Cracking the Code: Metadata Problems in Media and Entertainment
Metadata Problems in Media and Entertainment is often the unsung hero in the media and entertainment industry. Whether it’s a blockbuster movie, your favorite TV series, or a music album, metadata ensures that content is easily found, categorized, and delivered to the right audience.
It acts as the glue that holds together massive amounts of digital content, helping media companies manage and distribute their libraries efficiently.
However, the journey isn’t always smooth. In today’s fast-paced digital world, metadata management has hit a few speed bumps, making it one of the most overlooked challenges in the media industry.
The problem is complex: as content grows, so does the volume and complexity of its metadata. Without proper management, this can lead to misclassification, poor discoverability, and even lost revenue.
In this post, we’ll unravel the common metadata issues in media and entertainment, highlight how these problems affect the industry, and explore possible solutions that can save time, effort, and money.

Metadata: The Backbone of Content Discovery
Metadata is more than just a technical term. In the context of media and entertainment, it’s the backbone of content discovery. In simple terms, metadata refers to the “data about data” that tells us what a piece of content is, who made it, and where it belongs.
It includes critical information like titles, genres, cast and crew names, and much more.
Imagine trying to find a movie without any search information like the title, cast, or genre—it’s nearly impossible, right? Metadata allows users to search and discover content across multiple platforms, be it a streaming service or a digital library.
Despite its importance, managing metadata efficiently is a challenge. With each new piece of content comes more metadata, and with that, the potential for numerous problems.
The Complex Nature of Media Metadata
Managing metadata is far from simple, especially in the media and entertainment industry, where there’s an overwhelming variety of content formats and platforms. One of the biggest challenges is the sheer diversity of metadata.
Metadata isn’t just a simple tag; it includes everything from technical specifications (such as file size and format) to creative descriptions (such as plot summaries, episode titles, and cast lists).
Consider a TV show with hundreds of episodes, each needing its own metadata—such as title, synopsis, and keywords. The more content there is, the more metadata you have to manage, leading to an exponentially growing challenge.
This vast complexity can quickly become a nightmare for media companies, who must manage this data across multiple platforms, ensuring it remains consistent, accurate, and up to date.
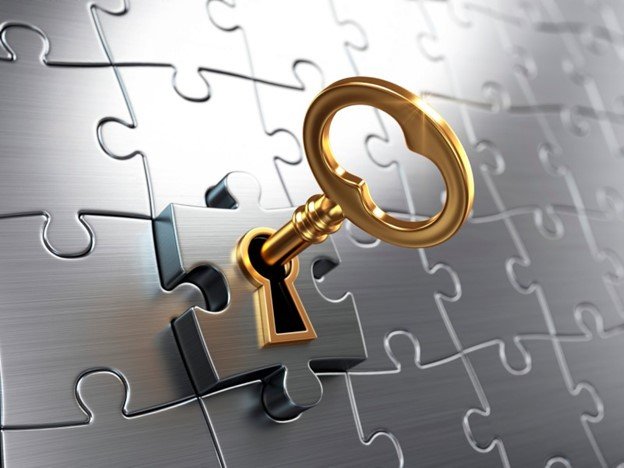
Automated Metadata: The Need for Speed
Given the complexity, the solution to this overwhelming challenge may seem obvious: automation. Automated metadata generation has become a popular tool for speeding up the process of tagging, categorizing, and keywording large amounts of content.
Streaming platforms, for example, use automated systems to generate metadata for their vast libraries.
Automated systems can save valuable time, especially when dealing with massive datasets. AI tools can analyze content and automatically generate relevant metadata—such as genres, themes, and even emotional tones.
However, relying solely on automated systems can backfire. AI systems, while fast, are not always accurate. This leads us to one of the biggest metadata problems in media: quality control.
AI Metadata Generation and Its Challenges
While AI can generate metadata at speeds that far outpace humans, speed doesn’t always mean accuracy. Automated metadata generation tools rely on algorithms, and these algorithms sometimes make mistakes.
An AI might incorrectly label a drama as a comedy or fail to capture important aspects of a piece of content, such as an actor’s performance or the tone of a particular scene.
For instance, imagine an AI mislabeling a suspense thriller as a romantic comedy. This error could lead to misdistribution, poor recommendations, and lost opportunities for viewer engagement.
Such misclassification isn’t just a small hiccup; it can have a real impact on content discoverability and monetization.
Furthermore, AI-generated metadata may miss out on more nuanced data points, such as cultural references or subtle emotional undertones that are better interpreted by human beings. This is where human oversight becomes crucial.
Human Oversight: Striking the Right Balance
No matter how advanced AI and machine learning systems become, there is still a critical need for human oversight in metadata management. Humans can add a level of understanding and context that machines cannot match.
We have the ability to pick up on subtle details, historical context, and cultural nuances that algorithms may overlook.
The real challenge is finding the right balance between automation and human intervention. Rely too much on automation, and you risk compromising the quality of your metadata.
However, over-relying on human oversight can significantly slow down the process, especially when dealing with vast amounts of data.
To strike the perfect balance, media companies must combine the speed of automation with the expertise of human insight. This approach ensures both efficiency and accuracy.
Metadata Quality Control: Ensuring Accuracy
To prevent metadata-related issues from spiraling out of control, media companies must invest in robust quality control measures. While automated systems can churn out metadata at scale, it’s important to have checks in place to ensure that the generated metadata is accurate, relevant, and up to date.
Without these quality controls, companies run the risk of errors such as incorrect categorization, outdated tags, or even incorrect language encoding. Such mistakes can cause big problems for content distribution and discovery.
One effective method for maintaining metadata quality is using a combination of automated checks and manual review. Automated systems can flag inconsistencies or potential errors, while human reviewers can step in to correct more complex or nuanced issues.
Machine Learning and Metadata Optimization
Machine learning plays a key role in improving metadata management over time. Instead of relying solely on predefined rules or human input, machine learning algorithms can analyze large datasets, identify patterns, and make adjustments to optimize metadata more efficiently.
These systems can learn from past mistakes and reduce the frequency of errors in future metadata generation. For instance, if a machine learning system identifies a recurring pattern of mislabeling certain types of content, it can adjust its algorithms to improve accuracy over time.
However, machine learning isn’t foolproof. While it can reduce the burden on human workers and improve efficiency, it’s still essential to have human intervention to address more complex issues or edge cases.
Metadata’s Role in Content Monetization
In the media and entertainment industry, metadata is more than just a technical necessity—it plays a direct role in content monetization. Accurate metadata ensures that content is easily discoverable, which leads to higher engagement, increased viewership, and ultimately, more revenue.
For example, imagine a new release buried deep within a streaming service’s library due to poor metadata. If viewers can’t find it, they won’t watch it, and the opportunity to generate revenue from that content disappears.
Good metadata is also crucial for targeted advertising and subscription-based recommendation algorithms. By ensuring that the right content is delivered to the right audience, media companies can maximize their revenue potential.

Addressing Common Metadata Problems
While the challenges of metadata management may seem overwhelming, they are not insurmountable. Many of the common problems—such as inconsistent naming conventions, missing tags, and outdated information—can be addressed with the right tools and processes.
For example, developing standard metadata guidelines across platforms can help ensure consistency. Likewise, investing in AI and machine learning tools can automate much of the metadata generation process, while human oversight can catch errors and ensure accuracy.
Metadata Standards: Why Consistency Matters
One of the biggest challenges in metadata management is maintaining consistency across different platforms, formats, and geographic locations. Inconsistent metadata can create significant problems for media companies, especially those distributing content globally. Variations in naming conventions, language encoding, and tag structures can lead to confusion, misclassification, and even legal complications.
For instance, a TV show might be labeled as “drama” in one country and “thriller” in another, leading to inconsistent categorization across platforms. Similarly, different language encodings might cause issues when the same content is distributed to international audiences. In the worst cases, inconsistent metadata could even lead to licensing violations, where content is mistakenly distributed in regions where it’s restricted.
To address these challenges, the media and entertainment industry has begun developing standardized metadata frameworks that aim to streamline the process.
Standards like the Entertainment Identifier Registry (EIDR) and Digital Object Identifier (DOI) systems are used to ensure consistency and uniformity across different platforms, but not all companies adhere to these standards.
Having industry-wide metadata standards is essential for future-proofing metadata management, especially as media companies look to expand their reach across multiple platforms and territories.
Future-Proofing Metadata Management
As the media landscape continues to evolve, metadata management will become even more critical. The rise of streaming platforms and the growing demand for digital content mean that metadata will continue to play a vital role in content discovery and monetization.
AI-driven automation and machine learning will play an increasingly important role in managing large-scale content libraries. However, metadata problems will likely persist as long as media companies rely on diverse systems and platforms. Also read this Sustainable Design at Open House Perth
The key to overcoming these challenges lies in a hybrid approach—balancing automation with human oversight, ensuring proper quality control, and continuously optimizing metadata management processes with machine learning.

While metadata may not always get the spotlight, its role in media and entertainment is essential. By addressing the ongoing challenges in metadata management, companies can ensure that their content remains discoverable, monetizable, and future-proof in an ever-evolving digital world.
What is metadata in media and entertainment?
Metadata is “data about data,” referring to information that describes content like titles, cast, genres, and keywords that help categorize and locate media in digital libraries.
Why is metadata important in the media industry?
Metadata ensures that content is searchable, discoverable, and categorized properly, making it crucial for content monetization and user engagement.
What are the common metadata problems in media and entertainment?
Common issues include inconsistent naming conventions, missing or incorrect tags, outdated information, and categorization errors, all of which can harm content discoverability and monetization.
How does AI help in metadata management?
AI automates metadata generation, speeding up the process and optimizing data through machine learning, though it still requires human oversight to ensure accuracy and contextual relevance.
How does metadata impact content monetization
Accurate metadata helps content get discovered by the right audience, driving engagement, viewership, and revenue, while poor metadata can lead to lost monetization opportunities.
Post Comment